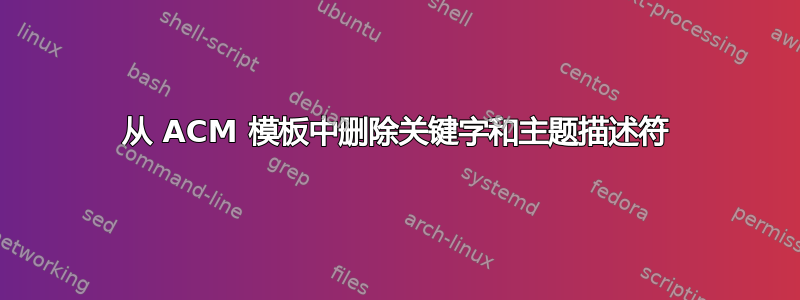
我正在尝试自定义 ACM 模板,即大格式单列模板[1]我真正想要的是摆脱的是主题描述和关键词部分。我知道它们在期刊中有多重要,但如果我想将模板用于其他用途,那么能够删除它们就太好了。在源代码中.tex
,它出现在摘要下,如下所示:
\end{abstract}
\category{H.5.2}{Information Interfaces and Presentation}{User
Interfaces}[Evaluation/\break methodology]
\category{H.1.2}{Models and Principles}{User/Machine Systems}[Human Information Processing]
\category{I.5.1}{Pattern\break Recognition}{Models}[Neural Nets]
\terms{Human Factors}
\keywords{Contour perception, flow visualization, perceptual theory, visual cortex, visualization}
\acmformat{Daniel Pineo, Colin Ware, and Sean Fogarty. 2010. Neural Modeling of Flow Rendering Effectiveness.}
如果我删除其中任何部分,所有摘要部分都会消失,我意识到文件.cls
需要编辑,但我确定需要编辑哪些部分。我真的很感激有人能指导我在哪里可以找到编辑它并删除类别和关键字部分。谢谢帮助!!
答案1
一些方式的改变\maketitle
需要对类定义的不是但是,修改原始.cls
文件;您可以在文档中使用重新定义,就像我在下面的示例代码中所做的那样(请注意,命令之间.tex
的部分),或者复制,调用它并替换\makeatletter
\makeatother
acmlarge.cls
myacmlarge.cls
\maketitle
用我的示例定义替换副本中的定义(没有在本例中使用\makeatletter
和\makeatother
);将副本保存在本地 TDS 树或当前工作目录中,然后使用
\documentclass[prodmode,acmtap]{myacmlarge}
在您的文档中。
示例代码:
\documentclass[prodmode,acmtap]{acmlarge}
\acmVolume{2}
\acmNumber{3}
\acmArticle{1}
\articleSeq{1}
\acmYear{2010}
\acmMonth{5}
\makeatletter
\def\maketitle{\newpage \thispagestyle{titlepage}\par
\begingroup \lineskip = \z@\null \vskip -13.5pt\relax
\parindent\z@ {\hyphenpenalty\@M
{\titlefont \@title \par
\global\firstfoot
\global\runningfoot
}}
\global\@firstpg\the\c@page
{\vskip 13.5pt\relax \normalsize \authorfont %vskip 13.5pt between title and author
\begingroup \addtolength{\baselineskip}{2pt}
\@author\par \vskip -2pt
\endgroup }
{%\ifx \@categories\@empty
%\else
\baselineskip 17pt\relax
\hbox{\vrule height .2pt width \@acmWidth}%to eliminate the lines for jacm
}
\vskip 8.5pt \footnotesize \box\@abstract \vskip 4pt\relax %vskip8.5 space above abstract
{\def\and{\unskip\/{\rm ; }}
% Categories and Subject Descriptors: \@categories \fi
}\par\vskip 4pt\relax
%\box\@terms \vskip 4pt\relax
%\box\@keywords \par
\ifx\@acmformat\@empty\else
\footnotesize \hsize \@acmWidth \parindent 0pt \noindent
\vskip 4\p@
\noindent {\bf ACM Reference Format:}\\[2pt]
\@acmformat\vskip 0.5\p@
\par\fi%
{\baselineskip 14pt\relax
\@abstractbottom
}
\vskip 23pt\relax
\endgroup
\let\maketitle\relax
\gdef\@categories{}}
%
\newbox\@abstract
\newbox\@terms
\newbox\@keywords
\def\abstract{\global\setbox\@abstract=\vbox\bgroup \everypar{}
%
\footnotesize \hsize \@acmWidth \parindent 10pt \noindent
\rule{0pt}{10pt}\ignorespaces}
\def\endabstract{\egroup}
\makeatother
% Title portion
\title{Neural Modeling of Flow Rendering Effectiveness}
\author{DANIEL PINEO and COLIN WARE \affil{University of New Hampshire}
SEAN FOGARTY
\affil{University of Illinois at Urbana-Champaign}
}
\begin{abstract}
It has been previously proposed that understanding the mechanisms of
contour perception can provide a theory for why some flow-rendering
methods allow for better judgments of advection pathways than others.
In the present article, we develop this theory through a numerical
model of the primary visual cortex of the brain (Visual Area 1) where
contour enhancement is understood to occur according to most
neurological theories. We apply a two-stage model of contour
perception to various visual representations of flow fields evaluated
using the advection task of Laidlaw et al. [2001]. In the first
stage, contour {enhancement} is modeled based on Li's cortical model
[Li 1998]. In the second stage, a model of streamline {tracing} is
proposed, designed to support the advection task. We examine the
predictive power of the model by comparing its performance to that of
human subjects on the advection task with four different
visualizations. The results show the same overall pattern for humans
and the model. In both cases, the best performance was obtained with
an aligned streamline-based method, which tied with a LIC-based
method. Using a regular or jittered grid of arrows produced worse
results. The model yields insights into the relative strengths of
different flow visualization methods for the task of visualizing
advection pathways.
\end{abstract}
\begin{document}
\maketitle
\section{Introduction}
\end{document}