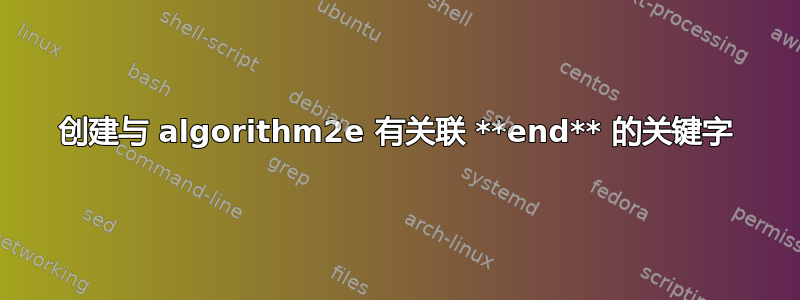
作为 Latex 课程家庭作业的一部分,我被要求用 Latex 编写该算法。
我已经用这段代码完成了大部分工作:-
\documentclass[12pt]{article}
\usepackage[margin=1in]{geometry}
\usepackage{graphicx, blindtext}
\usepackage{algorithm2e}
\usepackage{amsmath}
\begin{document}
\RestyleAlgo{boxed}
\begin{algorithm}[h!]
\DontPrintSemicolon
\SetKwInput{kwInput}{Input}
\SetKwInput{kwInit}{Initialization}
\SetKwInput{kwMain}{Forward Inclusion}
\SetKwInput{kwOutput}{Output}
\kwInput{
\par
\vspace{0.25cm}
Training examples $ Z = \{(x_1 , y_1 ), . . . , (x_N , y_N )\} $, where $N = a + b$, of which $a$ examples have $y_i = 1$ and $b$ examples have $ y_i = −1 $\;
\vspace{0.25cm}
The number $M$ of weak classifiers to be combined.
}
\kwInit{
\par
\vspace{0.25cm}
\Indp{
$
w_i^{(0)}= \begin{cases}
\frac{1}{2a} & \text{$for\ those\ examples\ with\ y_i=1$} \\
\frac{1}{2b} & \text{$otherwise$}
\end{cases}
$
}
\vspace{0.25cm}
}
\kwMain{
\par
\vspace{0.25cm}
\For{m = 1, . . . , M}{
Choose optimal $h_m$ to minimize the weighted error\;
Choose $a_m$ according to (2.17)\;
Update $w_i^{(m)} \gets w_i^{(m-1)} e^{-y_ia_mh_m(x_i)}$ and normalize to $\sum_i{w_i^{(m)}} = 1$\;
}
\vspace{0.25cm}
}
\kwOutput{
\par
Classification Function: $H_M(x)$ as in (2.20)\;
Class Label Prediction: $\hat{y}(x) = sgn(H_M(x))$\;
}
\caption{AdaBoost Learning Algorithm [35]}
\end{algorithm}
\end{document}
但是,我无法创建新的关键字(例如\kwInit
具有自己关联的新关键字(如上面的代码所示)结尾,以及自动缩进的代码段。
作为 Latex 排版系统的新手,非常欢迎任何帮助。
作为参考,我的代码产生如下输出:-
答案1
只需替换\SetKwInput{word}
为\SetKwBlock{beginword}{endword}
。请参阅algorithm2e
手动的第 11.2 节第 35 页。此修改提供
\documentclass[12pt]{article}
\usepackage[margin=1in]{geometry}
\usepackage{graphicx, blindtext}
\usepackage{algorithm2e}
\usepackage{amsmath}
\begin{document}
\RestyleAlgo{boxed}
\begin{algorithm}[h!]
\DontPrintSemicolon
\SetKwInput{kwInput}{Input}
\SetKwBlock{kwInit}{Initialization}{end}
\SetKwBlock{kwMain}{Forward Inclusion}{end}
\SetKwInput{kwOutput}{Output}
\kwInput{
\par
\vspace{0.25cm}
Training examples $ Z = \{(x_1 , y_1 ), . . . , (x_N , y_N )\} $, where $N = a + b$, of which $a$ examples have $y_i = 1$ and $b$ examples have $ y_i = −1 $\;
\vspace{0.25cm}
The number $M$ of weak classifiers to be combined.
}
\kwInit{
\par
\vspace{0.25cm}
\Indp{
$
w_i^{(0)}= \begin{cases}
\frac{1}{2a} & \text{$for\ those\ examples\ with\ y_i=1$} \\
\frac{1}{2b} & \text{$otherwise$}
\end{cases}
$
}
\vspace{0.25cm}
}
\kwMain{
\par
\vspace{0.25cm}
\For{m = 1, . . . , M}{
Choose optimal $h_m$ to minimize the weighted error\;
Choose $a_m$ according to (2.17)\;
Update $w_i^{(m)} \gets w_i^{(m-1)} e^{-y_ia_mh_m(x_i)}$ and normalize to $\sum_i{w_i^{(m)}} = 1$\;
}
\vspace{0.25cm}
}
\kwOutput{
\par
Classification Function: $H_M(x)$ as in (2.20)\;
Class Label Prediction: $\hat{y}(x) = sgn(H_M(x))$\;
}
\caption{AdaBoost Learning Algorithm [35]}
\end{algorithm}
\end{document}