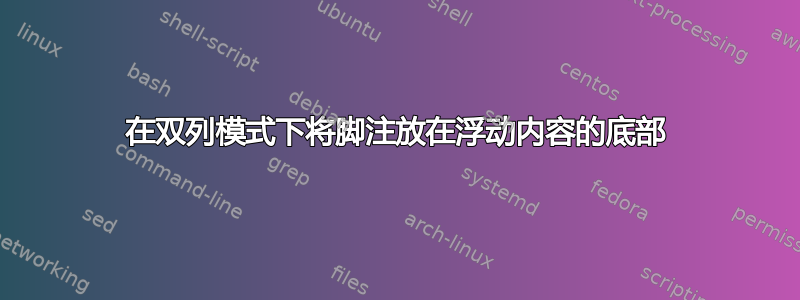
如何在模式下自动将脚注放在浮动底部twocolumn
?stfloats.sty
仅适用于单列文档,您能提供解决方案吗?请查看下面放置的我的 MWE:
\documentclass[twocolumn]{book}
\usepackage{stfloats}
\fnbelowfloat
\begin{document}
The traceability is of paramount importance and considered as a prerequisite for businesses for long-term functioning in today’s global supply chain. The implementation of traceability can create visibility by the systematic recall of information related to all processes and logistics movement. The traceability coding tag consists of unique features for identification, which links the product with traceability information, plays an important part in the traceability system. In this paper, we describe an innovative technique of product component-based traceability which demonstrates that product’s inherent features—extracted using deep learning—can be used as a traceability signature. This has been demonstrated on textile fabrics, where Faster region-based convolutional neural network (Faster R-CNN) has been introduced with transfer learning to provide a robust end-to-end solution for coded yarn recognition. The experimental results show that the deep learning-based algorithm is promising in coded yarn recognition, which indicates the feasibility for industrial application.\footnote{The traceability is of paramount importance and considered as a prerequisite for businesses for long-term functioning in today’s global supply chain.} The experimental results show that the deep learning-based algorithm is promising in coded yarn recognition, which indicates the feasibility for industrial application.\footnote{The traceability is of paramount importance and considered as a prerequisite for businesses for long-term functioning in today’s global supply chain.}
\begin{table*}[!b]
\caption{Specifications of coded yarn classes.}
{\tabcolsep0pt\begin{tabular*}{480pt}{@{\extracolsep{\fill}}@{}ccccccccccc@{}}
\hline
Represented digit & \multicolumn{2}{c}{0} & \multicolumn{2}{c}{1} & \multicolumn{2}{c}{2} & \multicolumn{2}{c}{3} & \multicolumn{2}{c}{4} \\
No. of twists per meter & \multicolumn{2}{c}{200} & \multicolumn{2}{c}{200} & \multicolumn{2}{c}{400} & \multicolumn{2}{c}{400} & \multicolumn{2}{c}{800} \\
Color composition & 2 White & 1 Black & 1 White & 2 Black & 2 White & 1 Black & 1 White & 2 Black & 2 White & 1 Black \\
\hline
\end{tabular*}}{}\end{table*}
The traceability is of paramount importance and considered as a prerequisite for businesses for long-term functioning in today’s global supply chain. The implementation of traceability can create visibility by the systematic recall of information related to all processes and logistics movement. The traceability coding tag consists of unique features for identification, which links the product with traceability information, plays an important part in the traceability system. In this paper, we describe an innovative technique of product component-based traceability which demonstrates that product’s inherent features—extracted using deep learning—can be used as a traceability signature. This has been demonstrated on textile fabrics, where Faster region-based convolutional neural network (Faster R-CNN) has been introduced with transfer learning to provide a robust end-to-end solution for coded yarn recognition. The experimental results show that the deep learning-based algorithm is promising in coded yarn recognition, which indicates the feasibility for industrial application.
The traceability is of paramount importance and considered as a prerequisite for businesses for long-term functioning in today’s global supply chain. The implementation of traceability can create visibility by the systematic recall of information related to all processes and logistics movement. The traceability coding tag consists of unique features for identification, which links the product with traceability information, plays an important part in the traceability system. In this paper, we describe an innovative technique of product component-based traceability which demonstrates that product’s inherent features—extracted using deep learning—can be used as a traceability signature. This has been demonstrated on textile fabrics, where Faster region-based convolutional neural network (Faster R-CNN) has been introduced with transfer learning to provide a robust end-to-end solution for coded yarn recognition.
The traceability is of paramount importance and considered as a prerequisite for businesses for long-term functioning in today’s global supply chain. The implementation of traceability can create visibility by the systematic recall of information related to all processes and logistics movement. The traceability coding tag consists of unique features for identification, which links the product with traceability information, plays an important part in the traceability system. In this paper, we describe an innovative technique of product component-based traceability which demonstrates that product’s inherent features—extracted using deep learning—can be used as a traceability signature. This has been demonstrated on textile fabrics, where Faster region-based convolutional neural network (Faster R-CNN) has been introduced with transfer learning to provide a robust end-to-end solution for coded yarn recognition. The experimental results show that the deep learning-based algorithm is promising in coded yarn recognition, which indicates the feasibility for industrial application.
\end{document}