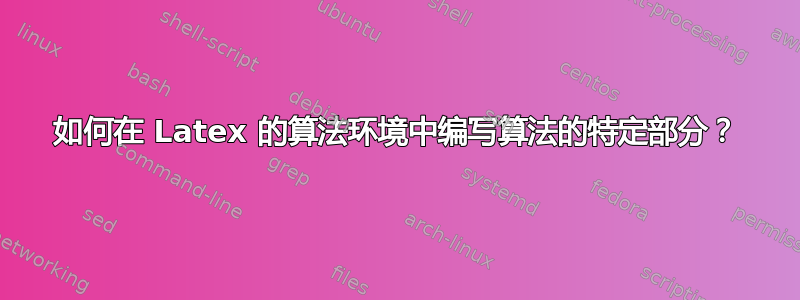
我能做的是这样的:
\documentclass{article}
\usepackage{algorithm}
\usepackage{algpseudocode}
\begin{document}
\begin{algorithm}
\caption{Optimizes a convex combination of $K$ kernels and employs a linear programming solver to iteratively solve the semi-infinite linear optimization problem \eqref{eq:sonnenburg2006large-12}.
The accuracy parameter $\varepsilon_{M K L}$ is a parameter of the algorithm.
$S_k(\alpha)$ and $c$ are determined by the cost function.
}
\begin{algorithmic}
\State $S^0=1, \theta^1=-\infty, \beta_k^1=\frac{1}{K}$ for $k=1, \ldots, K$
\For{$t=1,2, \ldots$}
\State Compute $\alpha^t=\underset{\alpha \in \mathcal{C}}{\operatorname{argmin}} \sum_{k=1}^K \beta_k^t S_k(\alpha)$ by single kernel algorithm with $\mathbf{k}=\sum_{k=1}^K \beta_k^t \mathbf{k}_k$
\State $S^t=\sum_{k=1}^K \beta_k^t S_k^t$, where $S_k^t=S_k\left(\alpha^t\right)$
\If{$\left|1-\frac{S^t}{\theta^t}\right| \leq \varepsilon_{M K L}$}
\State \textbf{break}
\EndIf
\State $\left(\beta^{t+1}, \theta^{t+1}\right)=\operatorname{argmax} \theta$
\State \;\;\;w.r.t.
\State \;\;\;s.t.
\begin{align*}
\text{w.r.t.} & \quad \beta \in \mathbb{R}^K, \theta \in \mathbb{R} \\
\text{s.t.} & \quad \mathbf{0} \leq \beta, \quad \sum_{k=1}^K \beta_k=1 \text { and } \sum_{k=1}^K \beta_k S_k^r \geq \theta \text { for } r=1, \ldots, t
\end{align*}
\EndFor
\end{algorithmic}
\end{algorithm}
\end{document}
答案1
\documentclass{article}
\usepackage{amsmath}
\usepackage{amssymb}
\usepackage{algorithm}
\usepackage{algpseudocode}
\begin{document}
\begin{algorithm}
\caption{Optimizes a convex combination of $K$ kernels and employs a linear programming solver to iteratively solve the semi-infinite linear optimization problem \eqref{eq:sonnenburg2006large-12}.
The accuracy parameter $\varepsilon_{M K L}$ is a parameter of the algorithm.
$S_k(\alpha)$ and $c$ are determined by the cost function.}
\begin{algorithmic}
\State $S^0=1, \theta^1=-\infty, \beta_k^1=\frac{1}{K}$ for $k=1, \ldots, K$
\For{$t=1,2, \ldots$}
\State Compute $\alpha^t=\underset{\alpha \in \mathcal{C}}{\operatorname{argmin}} \sum_{k=1}^K \beta_k^t S_k(\alpha)$ by single kernel algorithm with $\mathbf{k}=\sum_{k=1}^K \beta_k^t \mathbf{k}_k$
\State $S^t=\sum_{k=1}^K \beta_k^t S_k^t$, where $S_k^t=S_k\left(\alpha^t\right)$
\If{$\left|1-\frac{S^t}{\theta^t}\right| \leq \varepsilon_{M K L}$}
\State \textbf{break}
\EndIf
\State $\left(\beta^{t+1}, \theta^{t+1}\right)=\operatorname{argmax} \theta$
\begin{align*}
\text{w.r.t.} & \quad \beta \in \mathbb{R}^K, \theta \in \mathbb{R} \\
\text{s.t.} & \quad \mathbf{0} \leq \beta, \quad \sum_{k=1}^K \beta_k=1 \text { and } \sum_{k=1}^K \beta_k S_k^r \geq \theta \text { for } r=1, \ldots, t
\end{align*}
\EndFor
\end{algorithmic}
\end{algorithm}
\end{document}