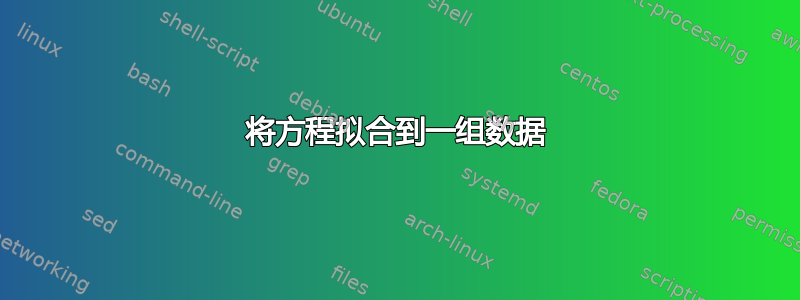
我有一组数据:
1.12158 0.42563 0.07
1.12471 0.42112 0.07
1.12784 0.41685 0.07
1.13097 0.41283 0.07
1.13409 0.40907 0.07
1.13722 0.40556 0.07
1.14035 0.40231 0.07
1.14348 0.39933 0.07
1.1466 0.39661 0.07
1.14973 0.39417 0.07
1.15285 0.39201 0.07
1.15598 0.39012 0.07
1.15911 0.38852 0.07
1.16224 0.3872 0.07
1.16536 0.38618 0.07
1.16849 0.38544 0.07
1.17162 0.385 0.07
1.17474 0.38486 0.07
1.17787 0.38543 0.07
1.181 0.38714 0.07
1.18413 0.38994 0.07
1.18725 0.39378 0.07
1.19038 0.39858 0.07
1.19351 0.40426 0.07
1.19664 0.41071 0.07
1.19976 0.41786 0.07
第一列是 x 轴,第二列是 y 轴。
我想将这些数据拟合到方程中:
Ax^2 + Bx + c
并找出 A、B 和 c 的值。
我可以使用什么程序?
如果您能告诉我如何做,我将非常高兴。
谢谢。
答案1
GNUplot:CLI 解决方案
假设data.dat
是包含数据的文件。
$ gnuplot
gnuplot> fit a*x**2 + b*x + c 'data.dat' via a, b, c
(...)
Final set of parameters Asymptotic Standard Error
======================= ==========================
a = 22.2174 +/- 1.09 (4.906%)
b = -51.7961 +/- 2.53 (4.885%)
c = 30.5745 +/- 1.468 (4.802%)
(...)
看适合文档中的部分以获得更多选择。
您还可以直接通过管道连接到 GNUPlot:
printf '%s\n' 'fit a*x**2 + b*x + c "data.dat" via a, b, c' | gnuplot
答案2
XMGrace(也称为 Grace):GUI 解决方案
假设data.dat
是包含数据的文件。
xmgrace data.dat
XMGrace 窗口会弹出,其中有一条代表数据的曲线。
在工具栏上,选择Data > Transformations > Regression
。选择Type of Fit: Quadratic
和Accept
。
将绘制一条新的拟合曲线,并弹出一个“控制台”:
(...)
y = 30.575 - 51.796 * x + 22.217 * x^2
(...)
您可以进一步使用 GUI 将数据制作为黑点并拟合为红色曲线。
XMGrace 还提供 CLI 界面,尽管其中缺少某些功能。您可以通过以下方式了解更多信息访问文档。
答案3
通过 CLI 在 R 中解决方案
Linux 中第一个终端类型R
然后
data.dat<-read.table(textConnection("a b c
1.12158 0.42563 0.07
1.12471 0.42112 0.07
1.12784 0.41685 0.07
1.13097 0.41283 0.07
1.13409 0.40907 0.07
1.13722 0.40556 0.07
1.14035 0.40231 0.07
1.14348 0.39933 0.07
1.1466 0.39661 0.07
1.14973 0.39417 0.07
1.15285 0.39201 0.07
1.15598 0.39012 0.07
1.15911 0.38852 0.07
1.16224 0.3872 0.07
1.16536 0.38618 0.07
1.16849 0.38544 0.07
1.17162 0.385 0.07
1.17474 0.38486 0.07
1.17787 0.38543 0.07
1.181 0.38714 0.07
1.18413 0.38994 0.07
1.18725 0.39378 0.07
1.19038 0.39858 0.07
1.19351 0.40426 0.07
1.19664 0.41071 0.07
1.19976 0.41786 0.07"),header=TRUE)
然后
plot(data.dat$a,data.dat$b,col="red",type="b")
为了解决使用以下
fit<-lm(data.dat$b~poly(data.dat$a,2,raw=TRUE))
summary(fit)
Call:
lm(formula = data.dat$b ~ poly(data.dat$a, 2, raw = TRUE))
Residuals:
Min 1Q Median 3Q Max
-0.0041754 -0.0021479 0.0004573 0.0019714 0.0059427
Coefficients:
Estimate Std. Error t value Pr(>|t|)
(Intercept) 30.575 1.468 20.83 < 2e-16 ***
poly(data.dat$a, 2, raw = TRUE)1 -51.796 2.530 -20.47 2.91e-16 ***
poly(data.dat$a, 2, raw = TRUE)2 22.217 1.090 20.38 3.20e-16 ***
---
Signif. codes: 0 ‘***’ 0.001 ‘**’ 0.01 ‘*’ 0.05 ‘.’ 0.1 ‘ ’ 1
Residual standard error: 0.002729 on 23 degrees of freedom
Multiple R-squared: 0.9568, Adjusted R-squared: 0.9531
F-statistic: 255 on 2 and 23 DF, p-value: < 2.2e-16