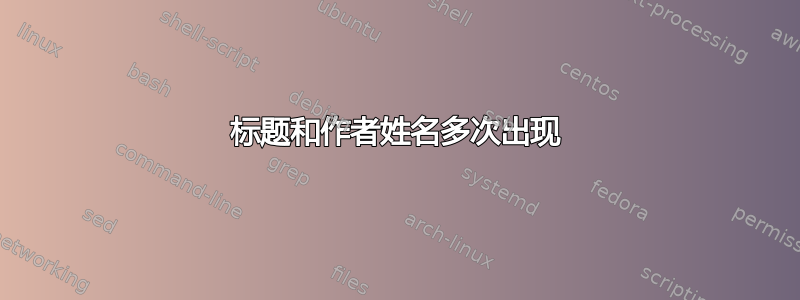
\documentclass[preprint,3p,times,twocolumn]{elsarticle}
\usepackage[version=3]{mhchem}
\usepackage{chemist}
\usepackage{unitsdef}
\usepackage{subfigure}
\usepackage{setspace}
\usepackage[utf8]{inputenc}
\doublespacing
\usepackage{amssymb}
\journal{Sensors and Actuators B}
\usepackage{etoolbox}
\pagestyle{plain}
\begin{document}
\title{\ce{LiFe_5O_8}-Based Gas Sensor: A Machine Learning Approach for Selective VOCs Detection}
\author[inst1]{DDDDDD}
\affiliation[inst1]{organization={School of Nano Science and Technology},%Department and Organization
addressline={XXXYYYY},
city={XXX},
postcode={88888},
state={West Bengal},
country={India}}
\author[inst2]{AAAAAA,\corref{cor1}}
\ead{[email protected]}
\author[inst1]{CCCCC}
\author[inst3]{SSSSSS}
\cortext[cor1]{Corresponding author}
\affiliation[inst2]{organization={Material Science Centre},
addressline={IPG},
city={Khoragpur},
postcode={88888},
state={West Bengal},
country={India}}
\affiliation[inst3]{organization={Department of Computer Science and Engineering},
addressline={IPG},
city={Khoragpur},
postcode={9999},
state={West Bengal},
country={India}}
\clearpage
\begin{frontmatter}
\begin{graphicalabstract}[ht]
\includegraphics{figures/abstractfig.png}
\end{graphicalabstract}
\begin{abstract}
Various characterization methods were also used to validate the material characteristics of the sensor.
The primary characterizations including XRD, FESEM, and XPS confirmed the formation of \ce{LiFe_{5}O_{8}} micro-flowers with an average size of 2.8 \micrometer without any impurities. This work presents the development of novel \ce{LiFe_{5}O_{8}} micro-flowers through a single-step microwave-assisted hydrothermal approach. \ce{LiFe_{5}O_{8}} micro-flowers were used as a sensing element for the detection of highly cross-sensitive volatile organic compounds (VOC). Various characterization methods were also used to validate the material characteristics of the sensor. The measured response/recovery curve revealed that these unique micro flowers can detect even at 5 ppm concentrations of acetone, ethanol, and methanol with response values of 1.17, 1.37, and 1.23 at 100\textdegree C respectively. The sensor’s selectivity issue was addressed by employing simpler Machine Learning (ML) algorithms (decision tree, random forest, K-nearest neighbour, and support vector machine) using the measured response values ranging from 5 ppm to 200 ppm. Further, the classification of the mixture and pure gases were performed using random mixture of the three VOCs to enhance the selectivity. The article compares various extracted features to determine the best feature set for the deployed ML algorithms. Optimization algorithms such as grid and randomized search were employed to identify the most suitable parameters for achieving the best models. The testing results reports an average classification accuracy of 98\% and an R2-score of 0.98 for the three gases from the best model.
\end{abstract}
\begin{highlights}
\item A novel \ce{LiFe_{5}O_{8}}-based VOC sensor is fabricated with good response value.
\item Selectivity of VOC sensor is improved by integrating ML; accurately identifies highly cross-sensitive VOC gases.
\item Different feature sets are explored to improve prediction performance.
\item Best training parameters are obtained with optimization algorithms for optimising the ML models.
\item Selectivity is further enhanced by classifying mixture and pure VOCs.
\end{highlights}
\begin{keyword}
Gas sensor \sep Hydro-thermal method \sep \ce{LiFe_5O_8} \sep Machine Learning \sep Selectivity \sep Volatile Organic Compounds
\end{keyword}
\end{frontmatter}
标题和作者姓名在图形摘要页面、突出显示页面和摘要页面上多次出现。我使用了类似的问题这里。上面说\pagestyle{plain}
在之前使用\begin{document}
,但并没有解决问题。任何帮助都将不胜感激。