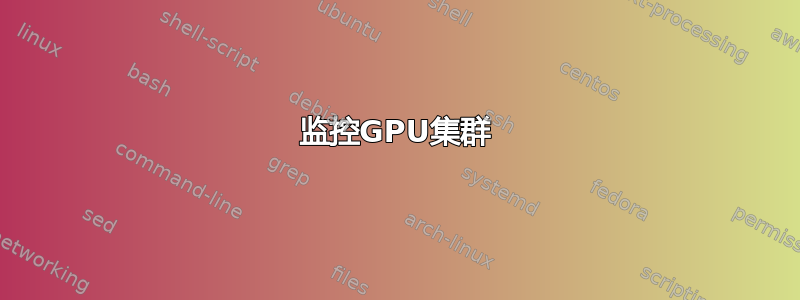
我有 10 台服务器在 Ubuntu 14.04 x64 上运行。每台服务器都有一些 Nvidia GPU。我正在寻找一个监控程序,可以让我一目了然地查看所有服务器上的 GPU 使用情况。
答案1
您可以使用神经节监控软件(免费、开源)。它有数量用户贡献的 Gmond Python DSO 度量模块,包括一个 GPU Nvidia 模块(/ganglia/gmond_python_modules/gpu/nvidia/
)。
其架构是典型的集群监控软件:
(图像来源)
安装起来很简单(大约 30 分钟,不着急),除了 GPU Nvidia 模块,它缺乏明确的文档。 (我仍然卡住)
要安装 ganglia,您可以执行以下操作。在服务器上:
sudo apt-get install -y ganglia-monitor rrdtool gmetad ganglia-webfrontend
Yes
每次询问有关 Apache 的问题时选择
第一的,我们配置 Ganglia 服务器,即gmetad
:
sudo cp /etc/ganglia-webfrontend/apache.conf /etc/apache2/sites-enabled/ganglia.conf
sudo nano /etc/ganglia/gmetad.conf
在 中gmetad.conf
,进行以下更改:
代替:
data_source "my cluster" localhost
通过(假设192.168.10.22
是服务器的IP)
data_source "my cluster" 50 192.168.10.22:8649
这意味着 Ganglia 应该监听 8649 端口(这是 Ganglia 的默认端口)。您应该确保 IP 和端口可供将在您计划监控的计算机上运行的 Ganglia 客户端访问。
您现在可以启动 Ganglia 服务器:
sudo /etc/init.d/gmetad restart
sudo /etc/init.d/apache2 restart
您可以访问网络界面http://192.168.10.22/ganglia/(其中192.168.10.22
是服务器的IP)
第二gmond
,我们在同一台机器或另一台机器上配置 Ganglia 客户端(即)。
sudo apt-get install -y ganglia-monitor
sudo nano /etc/ganglia/gmond.conf
在 中gmond.conf
,进行以下更改,以便 Ganglia 客户端(即 )gmond
指向服务器:
代替:
cluster {
name = "unspecified"
owner = "unspecified"
latlong = "unspecified"
url = "unspecified"
}
到
cluster {
name = "my cluster"
owner = "unspecified"
latlong = "unspecified"
url = "unspecified"
}
代替
udp_send_channel {
mcast_join = 239.2.11.71
port = 8649
ttl = 1
}
经过
udp_send_channel {
# mcast_join = 239.2.11.71
host = 192.168.10.22
port = 8649
ttl = 1
}
代替:
udp_recv_channel {
mcast_join = 239.2.11.71
port = 8649
bind = 239.2.11.71
}
到
udp_recv_channel {
# mcast_join = 239.2.11.71
port = 8649
# bind = 239.2.11.71
}
您现在可以启动 Ganglia 客户端:
sudo /etc/init.d/ganglia-monitor restart
它应该在 30 秒内出现在服务器上的 Ganglia Web 界面中(即http://192.168.10.22/ganglia/)。
由于gmond.conf
所有客户端的文件都是相同的,因此您可以在几秒钟内将神经节监控添加到新计算机上:
sudo apt-get install -y ganglia-monitor
wget http://somewebsite/gmond.conf # this gmond.conf is configured so that it points to the right ganglia server, as described above
sudo cp -f gmond.conf /etc/ganglia/gmond.conf
sudo /etc/init.d/ganglia-monitor restart
我使用了以下指南:
- http://www.ubuntugeek.com/install-ganglia-on-ubuntu-14-04-server-trusty-tahr.html
- https://www.digitalocean.com/community/tutorials/introduction-to-ganglia-on-ubuntu-14-04
gmond
用于在您要监视的所有服务器上启动或重新启动的 bash 脚本:
deploy.sh
:
#!/usr/bin/env bash
# Some useful resources:
# while read ip user pass; do : http://unix.stackexchange.com/questions/92664/how-to-deploy-programs-on-multiple-machines
# -o StrictHostKeyChecking=no: http://askubuntu.com/questions/180860/regarding-host-key-verification-failed
# -T: http://stackoverflow.com/questions/21659637/how-to-fix-sudo-no-tty-present-and-no-askpass-program-specified-error
# echo $pass |: http://stackoverflow.com/questions/11955298/use-sudo-with-password-as-parameter
# http://stackoverflow.com/questions/36805184/why-is-this-while-loop-not-looping
while read ip user pass <&3; do
echo $ip
sshpass -p "$pass" ssh $user@$ip -o StrictHostKeyChecking=no -T "
echo $pass | sudo -S sudo /etc/init.d/ganglia-monitor restart
"
echo 'done'
done 3<servers.txt
servers.txt
:
53.12.45.74 my_username my_password
54.12.45.74 my_username my_password
57.12.45.74 my_username my_password
Web 界面主页截图:
https://www.safaribooksonline.com/library/view/monitoring-with-ganglia/9781449330637/ch04.html给出了 Ganglia Web 界面的一个很好的概述:
答案2
答案3
作为卡斯说,我可以编写自己的工具,所以这里是(根本没有完善,但它有效。):
客户端(即GPU节点)
gpu_monitoring.sh
(假设监控网页的服务器IP为128.52.200.39
)
while true;
do
nvidia-smi --query-gpu=utilization.gpu,utilization.memory,memory.total,memory.free,memory.used --format=csv >> gpu_utilization.log;
python gpu_monitoring.py
sshpass -p 'my_password' scp -o StrictHostKeyChecking=no ./gpu_utilization_100.png [email protected]:/var/www/html/gpu_utilization_100_server1.png
sshpass -p 'my_password' scp -o StrictHostKeyChecking=no ./gpu_utilization_10000.png [email protected]:/var/www/html/gpu_utilization_10000_server1.png
sleep 10;
done
gpu_monitoring.py
:
'''
Monitor GPU use
'''
from __future__ import print_function
from __future__ import division
import numpy as np
import matplotlib
import os
matplotlib.use('Agg') # http://stackoverflow.com/questions/2801882/generating-a-png-with-matplotlib-when-display-is-undefined
import matplotlib.pyplot as plt
import time
import datetime
def get_current_milliseconds():
'''
http://stackoverflow.com/questions/5998245/get-current-time-in-milliseconds-in-python
'''
return(int(round(time.time() * 1000)))
def get_current_time_in_seconds():
'''
http://stackoverflow.com/questions/415511/how-to-get-current-time-in-python
'''
return(time.strftime("%Y-%m-%d_%H-%M-%S", time.gmtime()))
def get_current_time_in_miliseconds():
'''
http://stackoverflow.com/questions/5998245/get-current-time-in-milliseconds-in-python
'''
return(get_current_time_in_seconds() + '-' + str(datetime.datetime.now().microsecond))
def generate_plot(gpu_log_filepath, max_history_size, graph_filepath):
'''
'''
# Get data
history_size = 0
number_of_gpus = -1
gpu_utilization = []
gpu_utilization_one_timestep = []
for line_number, line in enumerate(reversed(open(gpu_log_filepath).readlines())): # http://stackoverflow.com/questions/2301789/read-a-file-in-reverse-order-using-python
if history_size > max_history_size: break
line = line.split(',')
if line[0].startswith('util') or len(gpu_utilization_one_timestep) == number_of_gpus:
if number_of_gpus == -1 and len(gpu_utilization_one_timestep) > 0:
number_of_gpus = len(gpu_utilization_one_timestep)
if len(gpu_utilization_one_timestep) == number_of_gpus:
gpu_utilization.append(list(reversed(gpu_utilization_one_timestep))) # reversed because since we read the log file from button to up, GPU order is reversed.
#print('gpu_utilization_one_timestep: {0}'.format(gpu_utilization_one_timestep))
history_size += 1
else: #len(gpu_utilization_one_timestep) <> number_of_gpus:
pass
#print('gpu_utilization_one_timestep: {0}'.format(gpu_utilization_one_timestep))
gpu_utilization_one_timestep = []
if line[0].startswith('util'): continue
try:
current_gpu_utilization = int(line[0].strip().replace(' %', ''))
except:
print('line: {0}'.format(line))
print('line_number: {0}'.format(line_number))
1/0
gpu_utilization_one_timestep.append(current_gpu_utilization)
# Plot graph
#print('gpu_utilization: {0}'.format(gpu_utilization))
gpu_utilization = np.array(list(reversed(gpu_utilization))) # We read the log backward, i.e., ante-chronological. We reverse again to get the chronological order.
#print('gpu_utilization.shape: {0}'.format(gpu_utilization.shape))
fig = plt.figure(1)
ax = fig.add_subplot(111)
ax.plot(range(gpu_utilization.shape[0]), gpu_utilization)
ax.set_title('GPU utilization over time ({0})'.format(get_current_time_in_miliseconds()))
ax.set_xlabel('Time')
ax.set_ylabel('GPU utilization (%)')
gpu_utilization_mean_per_gpu = np.mean(gpu_utilization, axis=0)
lgd = ax.legend( [ 'GPU {0} (avg {1})'.format(gpu_number, np.round(gpu_utilization_mean, 1)) for gpu_number, gpu_utilization_mean in zip(range(gpu_utilization.shape[1]), gpu_utilization_mean_per_gpu)]
, loc='center right', bbox_to_anchor=(1.45, 0.5))
plt.savefig(graph_filepath, dpi=300, format='png', bbox_inches='tight')
plt.close()
def main():
'''
This is the main function
'''
# Parameters
gpu_log_filepath = 'gpu_utilization.log'
max_history_size = 100
max_history_sizes =[100, 10000]
for max_history_size in max_history_sizes:
graph_filepath = 'gpu_utillization_{0}.png'.format(max_history_size)
generate_plot(gpu_log_filepath, max_history_size, graph_filepath)
if __name__ == "__main__":
main()
#cProfile.run('main()') # if you want to do some profiling
服务器端(即Web服务器)
gpu.html
:
<!DOCTYPE html>
<html>
<body>
<h2>gpu_utilization_server1.png</h2>
<img src="gpu_utilization_100_server1.png" alt="Mountain View" style="height:508px;"><img src="gpu_utilization_10000_server1.png" alt="Mountain View" style="height:508px;">
</body>
</html>
答案4
或者简单地使用
https://github.com/PatWie/cluster-smi
它的行为方式与终端完全相同nvidia-smi
,但收集集群中运行cluster-smi-node
.输出将是
+---------+------------------------+---------------------+----------+----------+
| Node | Gpu | Memory-Usage | Mem-Util | GPU-Util |
+---------+------------------------+---------------------+----------+----------+
| node-00 | 0: TITAN Xp | 3857MiB / 12189MiB | 31% | 0% |
| | 1: TITAN Xp | 11689MiB / 12189MiB | 95% | 0% |
| | 2: TITAN Xp | 10787MiB / 12189MiB | 88% | 0% |
| | 3: TITAN Xp | 10965MiB / 12189MiB | 89% | 100% |
+---------+------------------------+---------------------+----------+----------+
| node-01 | 0: TITAN Xp | 11667MiB / 12189MiB | 95% | 100% |
| | 1: TITAN Xp | 11667MiB / 12189MiB | 95% | 96% |
| | 2: TITAN Xp | 8497MiB / 12189MiB | 69% | 100% |
| | 3: TITAN Xp | 8499MiB / 12189MiB | 69% | 98% |
+---------+------------------------+---------------------+----------+----------+
| node-02 | 0: GeForce GTX 1080 Ti | 1447MiB / 11172MiB | 12% | 8% |
| | 1: GeForce GTX 1080 Ti | 1453MiB / 11172MiB | 13% | 99% |
| | 2: GeForce GTX 1080 Ti | 1673MiB / 11172MiB | 14% | 0% |
| | 3: GeForce GTX 1080 Ti | 6812MiB / 11172MiB | 60% | 36% |
+---------+------------------------+---------------------+----------+----------+
使用 3 个节点时。
它使用 NVML 直接读取这些值以提高效率。我建议不是nvidia-smi
按照其他答案中的建议解析 的输出。此外,您可以cluster-smi
使用Python+ZMQ来跟踪这些信息。