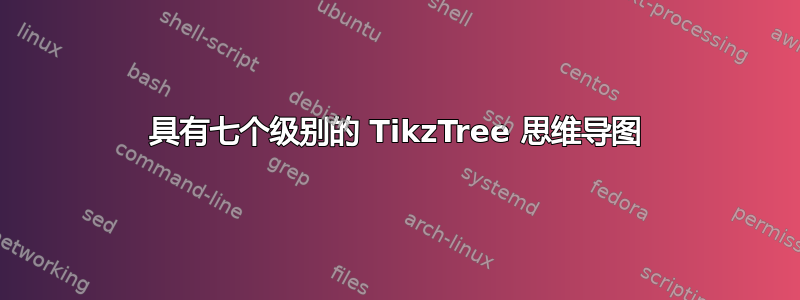
我想用 LaTeX 重新创建 860 个统计测试(假设检验)的思维导图。到目前为止,我找到了以下脚本:
\documentclass[border=10pt,multi,tikz]{standalone}
\usetikzlibrary{shapes.geometric}
\begin{document}
\tikzset{
treenode/.style = {draw,ellipse, align=left,
top color=white, bottom color=blue!20},
root/.style = {treenode, font=\large, bottom color=white},
env/.style = {treenode, font=\ttfamily\normalsize},
dummy/.style = {circle,draw}
}
\begin{tikzpicture}
[
level 1/.style={ edge from parent path={
(\tikzparentnode) to[out=0, in=180] (\tikzchildnode)
-- (\tikzchildnode)
},},
level 2/.style={minimum width=200pt, edge from parent path={
(\tikzparentnode.east) to[out=0, in=180] (\tikzchildnode.south west)
-- (\tikzchildnode.south east)
},
sibling distance=0.6cm},
level 1/.append style={level distance=4.5cm},
level 2/.append style={level distance=6.5cm},
%edge from parent/.style = {draw, -latex},
%every node/.style = {font=\footnotesize},
sloped,
]
\node [root] {Algorithm}
[grow=right]
child [sibling distance=4.0cm] { node{Clustering}
child { node {k-Means}}
child { node {k-Medians}}
child { node {Expectation Maximisation (EM)}}
child { node {Hierarchical Clustering}}
}
child [sibling distance=4.5cm] { node {Bayesian}
child { node {Naive Bayes}}
child { node {Gaussian Naive Bayes}}
child { node {Multinomial Naive Bayes}}
child { node {Averaged One-Depen. Esti. (AODE)}}
child { node {Bayesian Belief Network (BBN)}}
child { node {Bayesian Network (BN)}}
}
child [sibling distance=0.5cm] { node {Decision Tree}
child { node {Classification and Regression Tree (CART)}}
child { node {Iterative Dichotomiser 3 (ID3)}}
child { node {C4.5 and C5.0}}
child { node {Chi-squared Auto.Inte. Det. (CHAID)}}
child { node {Decision Stump}}
child { node {M5}}
child { node {Conditional Decision Trees}}
}
child [sibling distance=5.5cm]{ node {Dimesionality Reduction}
child { node {[Principal Component Analysis (PCA)}}
child { node {Principal Component Regression (PCR)}}
child { node {Partial Least Squares Regression\\(PLSR)}}
child { node {Sammon Mapping}}
child { node {Multidimensional Scaling (MDS)}}
child { node {Projection Pursuit}}
child { node {Linear Discriminant Analysis (LDA)}}
child { node {Mixture Discriminant Analysis (MDA)}}
child { node {Quadratic Discriminant Analysis (QDA)}}
child { node {Flexible Discriminant Analysis (FDA)}}
}
child [sibling distance=5.cm] { node {Regularisation}
child { node {Ridge Regression}}
child { node {Least Abs. Shr. and Sel. Ope. (LASSO)}}
child { node {Elastic Net}}
child { node {Least-Angle Regression (LARS)}}
};
\tikzset{
level 1/.style={ edge from parent path={
(\tikzparentnode) to[out=180, in=0] (\tikzchildnode)
-- (\tikzchildnode)
}},
level 2/.style={minimum width=200pt,align=left, edge from parent path={
(\tikzparentnode) to[out=180, in=0] (\tikzchildnode.south east)
-- (\tikzchildnode.south west)
},
sibling distance=0.6cm},
level 1/.append style={level distance=4.5cm},
level 2/.append style={level distance=5.5cm},
%edge from parent/.style = {draw, -latex},
%every node/.style = {font=\footnotesize},
sloped,
}
\node [root]{Algorithm}
[grow=left]
child [sibling distance=3.1cm]{ node{Deep Learning}
child { node {Deep Boltzmann Machine (DBM)}}
child { node {Deep Belief Network (DBN)}}
child { node {Convolutional Neural Network (CNN)}}
child { node {Stacked Auto-Encoders}}
}
child [sibling distance=2.8cm]{ node {Ensamble}
child { node {Boosting}}
child { node {Bootstrapped Aggregation (Bagging)}}
child { node {AdaBoost}}
child { node {Stacked Generalization (blending)}}
child { node {Gradient Boosting Machines (GBM)}}
child { node {Gradient Boosted Regr. Trees (GBRT)}}
child { node {Random Forest}}
}
child { node{Neural Networks}
child { node {Perceptron}}
child { node {Back-Propagation}}
child { node {Hopfield Network}}
child { node {Radial Basis Function Net. (RBFN)}}
}
child[sibling distance=3.9cm] { node {Insatnce Based}
child { node {k-Nearest Neighbour(KNN)}}
child { node {Learning vector quantization(LVQ)}}
child { node {self-organised map}}
child { node {Locally Weighted Learning(LWL)}}
}
child[sibling distance=3.2cm] { node {Rule system}
child { node {Cubist}}
child { node {One Rule (OneR)}}
child { node {Zero Rule (ZeroR)}}
child { node {Rep.Inc.Pr2pro. Err. Red. (RIPPER)}}
}
child [sibling distance=3.3cm]{ node {Regression}
child { node {Linear}}
child { node {Ordinary Least Squares}}
child { node {Logistic}}
child { node {Stepwise}}
child { node {Locally Esti.Scatt. Smoo.(LOESS)}}
child { node {Multi. Ada. Reg.Splines (MARS)}}
};
\end{tikzpicture}
\end{document}
得出:
但是我在添加第 3 级时已经卡住了,这样看起来就好了。我甚至无法理解第 3 级的机制,所以我已经知道我也会在第 4、5、6 和 7 级遇到问题。
任何帮助是极大的赞赏。
答案1
一些指导。
pgfmanual 中的最后一个教程涉及思维导图但对于初学者来说,这可不是一顿轻松的饭菜Tikz
。我宁愿让你注意第21章 让树木生长,如果需要的话,树木图书馆以下内容以第 21 章为指导。
我给你看的是:
- 右节点,向右生长一棵树
- 左节点也一样
- 最后在中间再画一个(例如,你可以事先将其分别放置在(0,0)处)
只要您设法遵循语法,child{
node {text}
}
您就可以继续前进;频繁编译有助于阻止错误(通常是括号)传播。
如您所见,原则上您可以扩展到(许多)任何级别。但很快您就会遇到定位问题。这就是级别特定的格式/样式发挥作用的地方,它允许您定位特定子级别的成员。请参阅第 21.4 章。
\documentclass[10pt,border=3mm,tikz]{standalone}
\begin{document}
\begin{tikzpicture}
% ~~~ a tree to the right ~~~~~~
\node (R) at (2,0) {root-R} [grow=right]
child{node {1-1}
child{node {2-1}}
child{node {2-2}
child{node {3-1}}
child{node {3-2}
}% closes 3-2
}% closes 2-2
}% closes 1-1
child{node {1-2}
child{node {2-2}}
}
;
% ~~~ a tree to the left ~~~~~~
\node (L) at (-2,0) {root-L} [grow=left]
child{node {1-1}}
child{node {1-2}}
;
% ~~~ let's draw sth. inbetween ~~~~
\draw (L) -- node[fill=white,draw=blue] {roots root} (R);
\end{tikzpicture}
\end{document}
你贴出的代码基本都是这个意思,把一些定位控制权交给了一些子元素,在语句中总结了很多与外观相关的样式\tikzset{}
。
我建议从小处着手,即“从文本外观和布局点缀方面进行裸露”,然后逐步引入一级又一级。